Congratulations Grace!
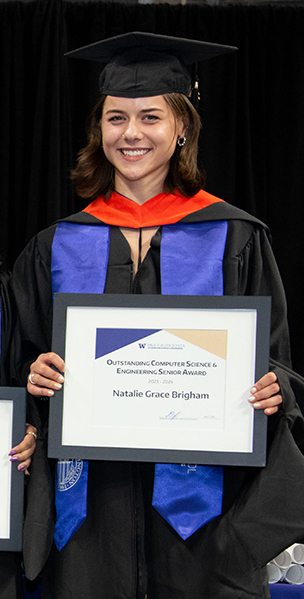
Huge congratulations to Security Lab MS graduate Grace Brigham, who received multiple awards at this year’s graduation: in addition to an Outstanding Senior Award, she was honored with the Outstanding Master’s Thesis Award for “Violation of my body: Perceptions of non-consensual (intimate) imagery.” That work, which Brigham completed under the supervision of Security Lab professor Tadayoshi Kohno, provided new insights into people’s perceptions of AI-generated non-consensual imagery that are already being used to inform national and international conversations about how to mitigate harms associated with such use of AI. The paper will be presented at the 20th Symposium on Usable Privacy and Security (SOUPS 2024).
(Details and photo from Allen School News, photo by Kerry Dahlen.)